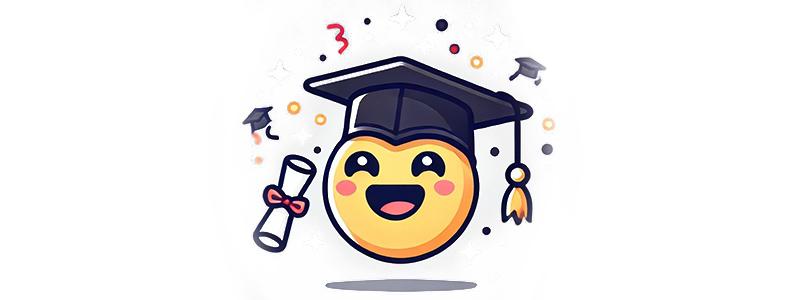
Knowledge Generation in Visual Analytics: Integrating Human and Machine Intelligence for Exploration of Big Data
2018Data Mining Dissertation
Big data poses many facets and challenges when analyzing data, often described with the five big V’s of Volume, Variety, Velocity, Veracity, and Value. However, the most important V – Value can only be achieved when knowledge can be derived from the data. The volume of nowadays datasets makes a manual investigation of all data records impossible and automated analysis techniques from data mining or machine learning often cannot be applied in a fully automated fashion to solve many real-world analysis problems, and hence, need to be manually trained or adapted. Visual analytics aims to solve this problem with a “human-in-the-loop” approach that provides the analyst with a visual interface that tightly integrates automated analysis techniques with human interaction. However, a holistic understanding of these analytic processes is currently an under-explored research area. A major contribution of this dissertation is a conceptual model-driven approach to visual analytics that focuses on the human-machine interplay during knowledge generation. At its core, it presents the knowledge generation model which is subsequently specialized for human analytic behavior, visual interactive machine learning, and dimensionality reduction. These conceptual processes extend and combine existing conceptual works that aim to establish a theoretical foundation for visual analytics. In addition, this dissertation contributes novel methods to investigate and support human knowledge generation processes, such as semi-automation and recommendation, analytic behavior and trust-building, or visual interaction with machine learning. These methods are investigated in close collaboration with real experts from different application domains (such as soccer analysis, linguistic intonation research, and criminal intelligence analysis) and hence, different data characteristics (geospatial movement, time series, and high-dimensional). The results demonstrate that this conceptual approach leads to novel, more tightly integrated, methods that support the analyst in knowledge generation. In a final broader discussion, this dissertation reflects the conceptual and methodological contributions and enumerates research areas at the intersection of data mining, machine learning, visualization, and human-computer interaction research, with the ultimate goal to make big data exploration more effective, efficient, and transparent.
Related Publication



