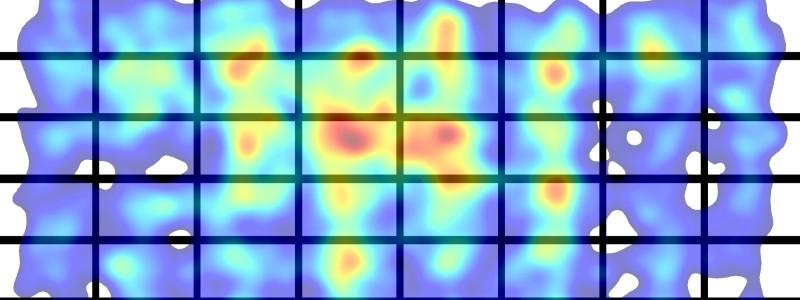
Known-Item Search in Video: An Eye Tracking-Based Study
L. Joos, B. Jäckl, D. A. Keim, M. T. Fischer, L. Peska, J. Lokoč
ICMR '24: Proceedings of the 2024 International Conference on Multimedia Retrieval, DOI:10.1145/3652583.3658119, 2024Empirical Studies Multimedia Analysis
Deep learning has revolutionized multimedia retrieval, yet effectively searching within large video collections remains a complex challenge. This paper focuses on the design and evaluation of known-item search systems, leveraging the strengths of CLIP-based deep neural networks for ranking. At events like the Video Browser Showdown, these models have shown promise in effectively ranking the video frames. While ranking models can be pre-selected automatically based on a benchmark collection, the selection of an optimal browsing interface, crucial for refining top-ranked items, is complex and heavily influenced by user behavior. Our study addresses this by presenting an eye tracking-based analysis of user interaction with different image grid layouts. This approach offers novel insights into search patterns and user preferences, particularly examining the trade-off between displaying fewer but larger images versus more but smaller images. Our findings reveal a preference for grids with fewer images and detail how image similarity and grid position affect user search behavior. These results not only enhance our understanding of effective video retrieval interface design but also set the stage for future advancements in the field.
Related Publication
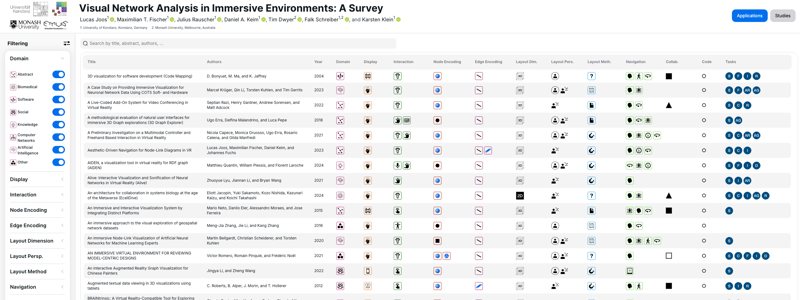
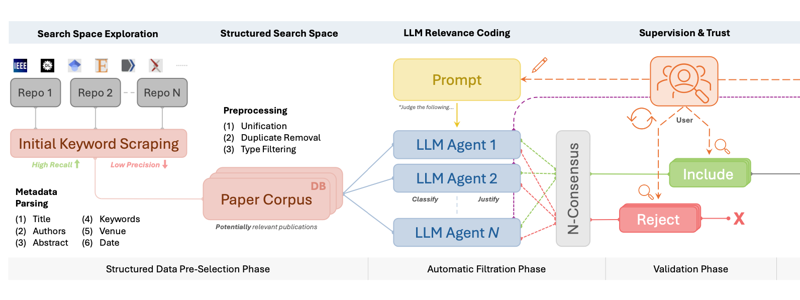
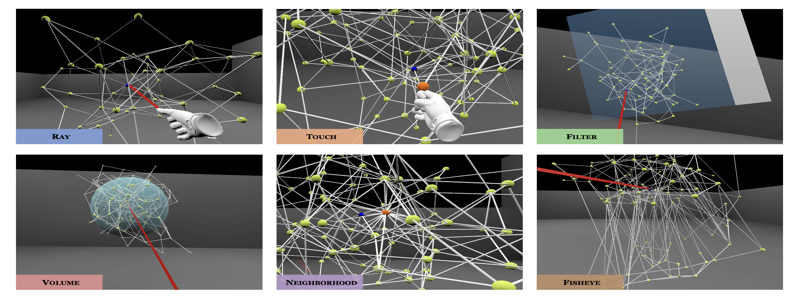
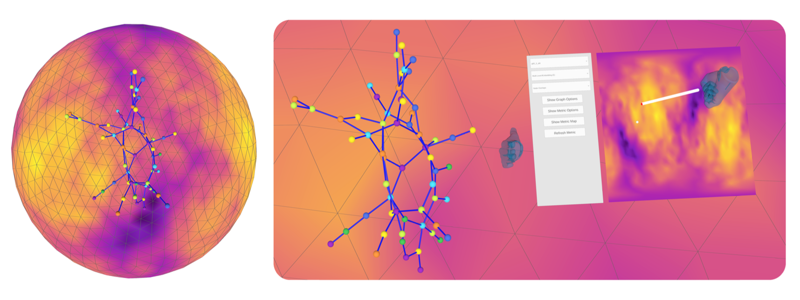
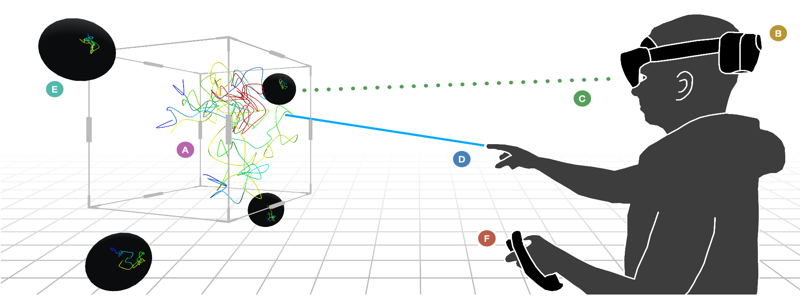